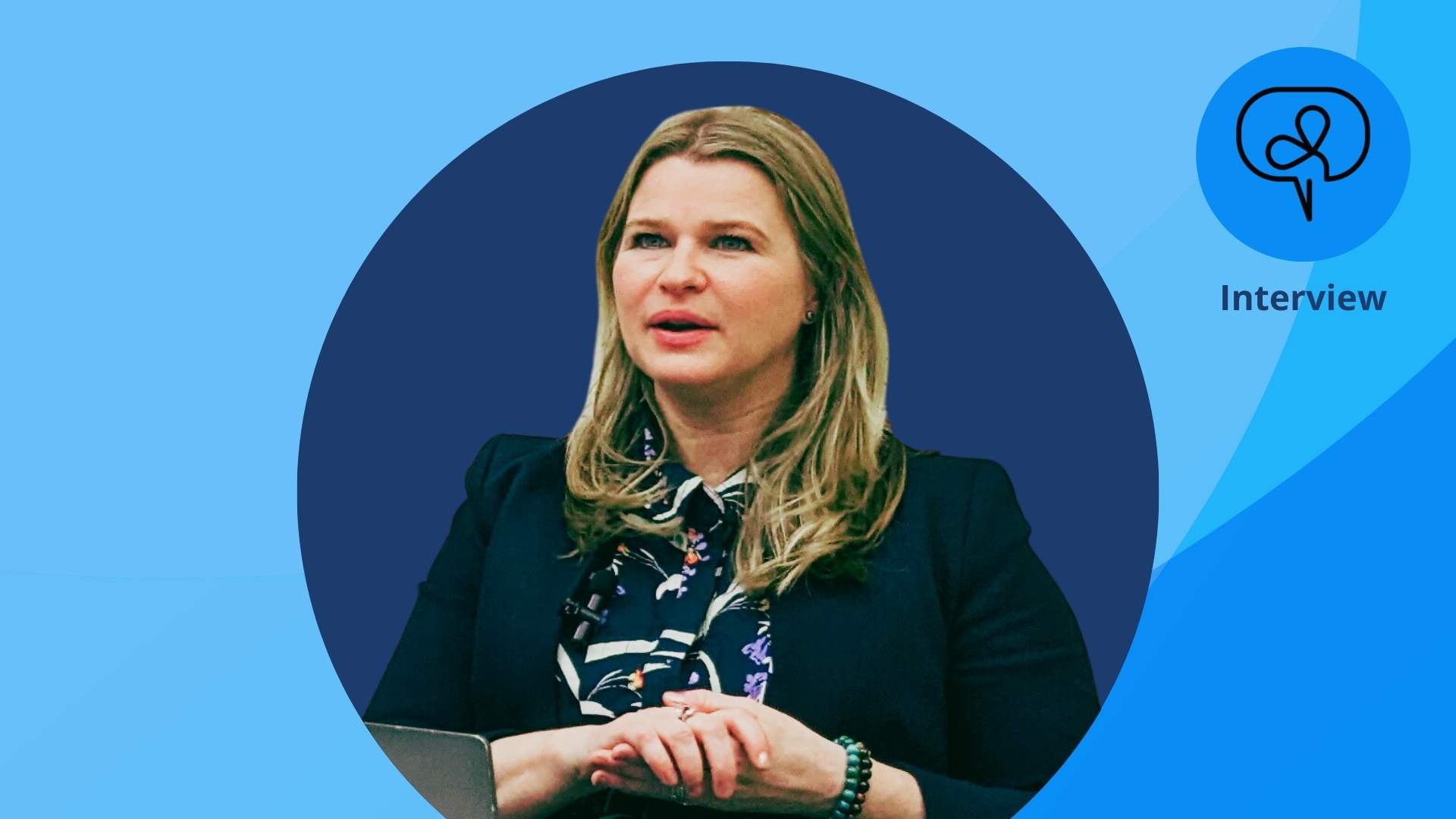
A conversation with Jaron Porciello, close partner of the GDPRD and member of the SDG2 Road Map Working Group.
What is the state of AI when it comes to transforming our food systems? Jaron Porciello shares her insights on the present and future of artificial intelligence in rural development.
Jaron Porciello is a Co-Director of Hesat2030 and an Associate Professor at the University of Notre Dame.
Secretariat/Maurizio Navarra: The past six months have been a whirlwind of news around artificial intelligence (AI) - the good, the bad, and the ugly. What can you tell us about the state of AI?
Jaron: The last six months have been a roller coaster. I like to joke that I start my day answering emails on all the great things about AI but end my day with those same questions but where people say, isn't this a doomsday prophecy? The truth is somewhere in between.
First the good. This has brought about phenomenal conversations between businesses, governments, different stakeholders, and the research community. They are having practical conversations about things like bias in machine learning models, the quality, quantity and availability of data, and data ownership. These are all things that my community of data, computer, and information scientists who are interested in ethical and responsible AI have been having for years!
There are great conversations about how to regulate this technology, which are long overdue. Large language models and in particular transformer models, have been around for more than five years. I use them every day in my work. What's different now is there's an application, such as the ChatGPT interface, for people to see how large language models work. It has taken what was working in the background for many programmes and brought it to the foreground.
What is not so good is the fear and rhetoric around the language of AI. An open letter “The future of AI” was put together by business leaders calling for a six months’ pause in the development of AI. If you think about it, that's a little crazy. How do you measure whether development and progress are moving too fast? We have to wonder if the call for a six-month hiatus is a chance for some businesses to catch up and figure out how to monetize new technologies? There are also hints that companies will look at artificial intelligence replacing human jobs, and the way this is being expressed in the media is causing fear and anxiety.
There are great conversations about how to regulate this technology, which are long overdue. Large language models and in particular transformer models, have been around for more than five years.
Maurizio: How could AI potentially change and have an impact on global food systems?
Jaron: Farmers were among the very first data scientists and have been recording data – temperature, precipitation, yield, pests, etc.—for thousands of years. And, agriculture was one of the first sectors to adopt AI technology. But because AI requires both data and large teams of computer and data scientists, many of the use cases of AI and its impact on global food systems are from the private sector/corporate world. For example, most modern tractors are equipped with remote sensors, which are constantly collecting localized and farm-level data, and which can be used to provide better intelligence to farmers and improve real time decisions. And AI helps large organizations like supermarkets and food distributors track food availability and food waste. With these use cases, I think some of the questions we need to keep asking about AI is in terms of corporate ownership. Who will own the different parts of this new technology, and the data associated with it? It is impossible to understate, however, the impact that new advances in AI will have on global food systems.
Meanwhile, I think it is important for the development community to have parallel conversation about the implications of AI and especially implications on small – scale farming communities. We are at the tip of the iceberg, especially in terms of use cases that take advantage of this technology to be used for global good without causing global harm.
Jaron Porciello | Hesat 2030 and University of Notre Dame. This video is from a recording of the interview, conducted by the Secretariat of the Global Donor Platform for Rural Development in May 2023.
Maurizio: What do you think AI means for international agencies and for the donor community?
Jaron: It’s an exciting time to figure out how to make better use of data that we already have. International agencies have rich data repositories, many of which are largely text-based. Now is the time for exploration and investigation to draw out more insights from these resources.
Agencies can play a leading role in guiding regulations of the technology to be equitable and inclusive, and also setting forward best practices for responsible AI. Many are familiar with the concept of a black box in AI, which refers to a model receiving an input, such as a question, and comes back with a decision or a recommendation, but it is not clear how the decision was made. What many may not realize is that AI doesn’t have to be a black box. Simple steps can be taken to show how model-based decisions can be presented transparently.
Agencies can also help in ensuring that government policies and regulations differentiate between different types of use cases and business models. While private sector and the public sector may be using the same technology base of large-language models, the use cases are different, and we need regulations that speak to how the private sector versus the public sector will use AI.
Regulating this technology will be one of the most difficult, but also one of the most important, challenges that policy makers have ever faced. We can’t run away from it, however – it is too important and has the potential to impact almost every aspect of our life.
A large language model is a series of mathematics and computation that looks to understand how humans use language. It looks at enormous repositories of text like Wikipedia and Google News, and learn, through a series of tasks, how humans put sentences together.
Maurizio: What are the challenges you face in working with artificial intelligence and large language models when it comes to working at the intersection of global, country, and local levels?
What should policy makers be thinking about when it comes to the regulations of these technologies?
Could you also provide a short explanation of large language models?
Jaron: A large language model is a series of mathematics and computation that looks to understand how humans use language. It looks at enormous repositories of text like Wikipedia and Google News, and learn, through a series of tasks, how humans put sentences together.
A language model has billions of different parameters. If you think about how a human speaks, we make jokes. We use expressions of love and hate, and of scientific and technical sophistication. A language model must think about how to learn all of that. And that's done by sequencing different data points that come together.
I see two main challenges working with across global, country, and regional levels.
One thing I think computer and data scientists often forget to communicate is that large-language models must be trained in order to be useful for specific tasks. In my work, I spend a lot of time working with experts to come up with concepts and datasets that can help train a model, identifying things like interventions, outcomes, populations, and other data points that are important for evidence-based decision making.
One challenge we face is the availability of data. We already know through 50 to 100 years of work that we have more published data coming from North America and Europe than from Africa and Southeast Asia. This implicitly biases a model in decision-making ability, especially if it is not properly trained. We know that context matters when looking for opportunities to work together to solve problems.
Languages are another challenge. Most models are trained on English-language materials. It's challenging to find models trained to work with non-English languages, especially local or regional languages, and this inherently biases what can be done with data models. The good news is that this issue is being taken seriously and I see concerted efforts to ensure that, where possible, models have looked at different languages and can tell you about the differences in what you can expect to achieve for any language.
Maurizio: You were a co-director of Ceres2030, a unique research project that presented a real evidence-based roadmap calling to double food-related aid to end hunger by 2030. Hesat2030 is the next phase. Could you tell us more about what Hesat2030 is and where it aims to take us next?
Jaron: Hesat2030: a global roadmap to end hunger sustainably and nutritiously is a new partnership driving change in global agrifood systems through better evidence, advocacy and innovation. We are led by the Food and Agriculture Organization of the United Nations (FAO), Shamba Centre for Food and Climate, and the University of Notre Dame and in partnership with CABI, Havos.Ai, Global Alliance for Improved Nutrition (GAIN); Global Donor Platform for Rural Development (GDPRD); International Food Policy Research Institute (IFPRI); and the University of Chicago. Hesat2030 was launched during the UN Food Systems Summit +2 Stocktaking Moment.
Hesat2030 will build on the evidence and costing of Ceres2030 by integrating additional outcomes, such as women’s empowerment, climate adaptation, and nutrition, to better understand how to improve the quality and quantity of official development assistance (ODA). We will be updating some of our global modelling figures, our evidence, and increasing outreach with the global community. We will continue to leverage advances in artificial intelligence and economic modelling. A global community of stakeholders is very important to the work of Hesat2030. The Donor Platform is a key stakeholder and community partner.
Ceres2030 was organized around a global modelling effort and a publication with Nature Research. With Hesat2030, we are publishing new findings more frequently while at the same time we work to update global modelling and a series of comprehensive recommendations by 2025.
We know that governments, funders, and stakeholder groups (including the Donor Platform’s SDG 2 Roadmap Working Group) need information faster. We want to increase efficiency in the global knowledge value chain, especially around science-policy information. Through our collaborations with groups like the Zero Hunger Coalition, Hesat2030 is producing a series of country-specific cost roadmaps for Madagascar, Zambia, and other countries. And the Juno Evidence Alliance will be releasing the State of the Field: Research in Agrifood Systems, which will use AI to look at more than 6 million papers in agrifood systems to better understand where we have high-quality evidence as well as how to identify complementary innovations from science faster.
The Platform's SDG2 Roadmap Working Group is a unique platform because we're able to receive feedback from donors on an ongoing basis. This better aligns our agendas with the messages donors are working on and ensures the evidence and cost modelling is time responsive to their concerns.
Maurizio: What gives you hope in 2023 and beyond?
Jaron: Communities are coming together to learn from each other. There is a willingness to work differently and to rely more on data and evidence. We're seeing incredible opportunities and commitments from groups working across the private sector, and groups convening farmer organizations and academics. Groups like the Juno Evidence Alliance and the Zero Hunger Coalition give me hope because they are bringing together a broad community of partners to ensure SDG2 is achieved by 2030.